Our article entitled “A systematic comparison of deep learning methods for Gleason grading and scoring“, J. P. Dominguez-Morales et al., has been published in Medical Image analysis.
Prostate cancer ranks second among men’s cancers globally, following lung cancer. Diagnosis relies on the Gleason score, assessing cell abnormality in glandular tissue through various patterns. Computational pathology advancements offer diverse datasets and algorithms for Gleason grading, yet consensus lacks on optimal methods matching data and labels.
This paper aims to guide researchers in selecting the most suitable practices based on the task and available labels. It systematically compares, on nine datasets, state-of-the-art training approaches for deep neural networks covering fully-supervised, weakly-supervised, semi-supervised, and various MIL methods. The results indicate that fully supervised learning is best suited for Gleason grading tasks, while Clustering-constrained Attention Multiple Instance Learning (CLAM) performs best for Gleason scoring.
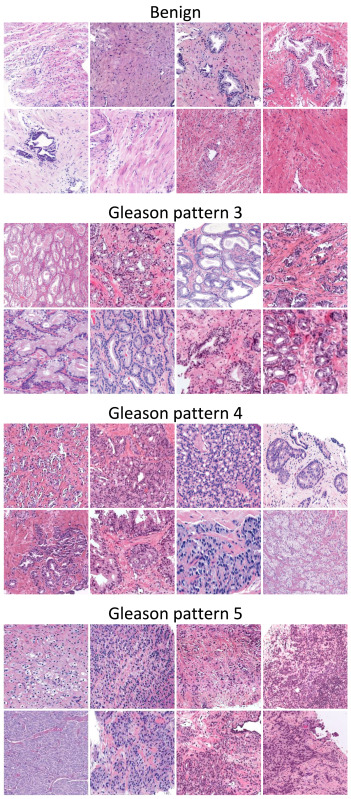