
Contact Details
Prof. Dr. Adrien Depeursinge University of Applied Sciences Western Switzerland (HES-SO)Institute of Information Systems
TechnoArk 3
CH-3960 Sierre, Valais, Switzerland
Tel +41 27 606 9023
Fax +41 27 606 9000
Email: adrien.depeursinge at hevs.ch
Publications
Projects
Research Interests
- Personalized medicine via image-based tissue analysis and machine learning for cancer and other diseases (Radiomics)
- Building digital models of disease-specific radiological phenotypes at the organ level using localization systems and texture-based computational models of biomedical tissue.
- N-dimensional texture analysis with control of scales and orientations
- Lung image analysis and retrieval (view video)
- Multimodal fusion
- Clinical workflows of image-based computer-aided systems
Modern multi-dimensional imaging in radiology yields much more information than the naked eye can appreciate. As a result, errors and variations in interpretations are currently the weakest aspect of clinical imaging. Computerized image analysis may provide solutions for ensuring the quality of medical image interpretation by yielding exhaustive, comprehensive and reproducible analysis of imaging features that are difficult to recognize with the naked eye.
We develop computational models of multi-dimensional morphological properties of biomedical tissue. Riesz wavelets and machine learning algorithms are used to learn the organization of image scales and directions that is specific to a given biomedical tissue type. The models obtained can be “steered” analytically to enable rotation-covariant image analysis. While most rotation-invariant approaches discard precious information about image directions, rotation-covariant analysis enables modeling the local organization of image directions independently from their global orientation. Although already being important in 2D images, this becomes crucial to adequately leverage directional image information in 3D images.
We evaluated and compared our approach to other state-of-the-art methods using benchmark collections of non-medical natural textures. Experimental evaluation reveals high classification accuracies for even orders of the Riesz transform, and suggests high robustness to changes in global image orientation and illumination. Overall, the proposed computational models were able to fit a wide range of textures and tissue structures. They can be trained to segment, classify and retrieve desired tissue categories. We are currently extending the framework to 3D, which is expected to fully leverage the wealth of modern radiology images.
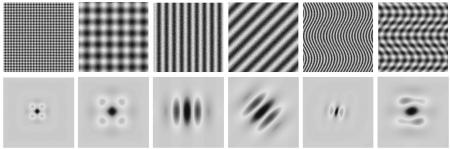

Fig.1. Example of multiscale texture signature learning using the Riesz transform (matlab code available!).
Fig.2. Building digital models of disease-specific radiological phenotypes of interstitial lung diseases.
Databases
A multimedia database of high-resolution computed tomography (HRCT) images from patients affected with interstitial lung diseases (ILD) is publicly available!